RapidRead Technology
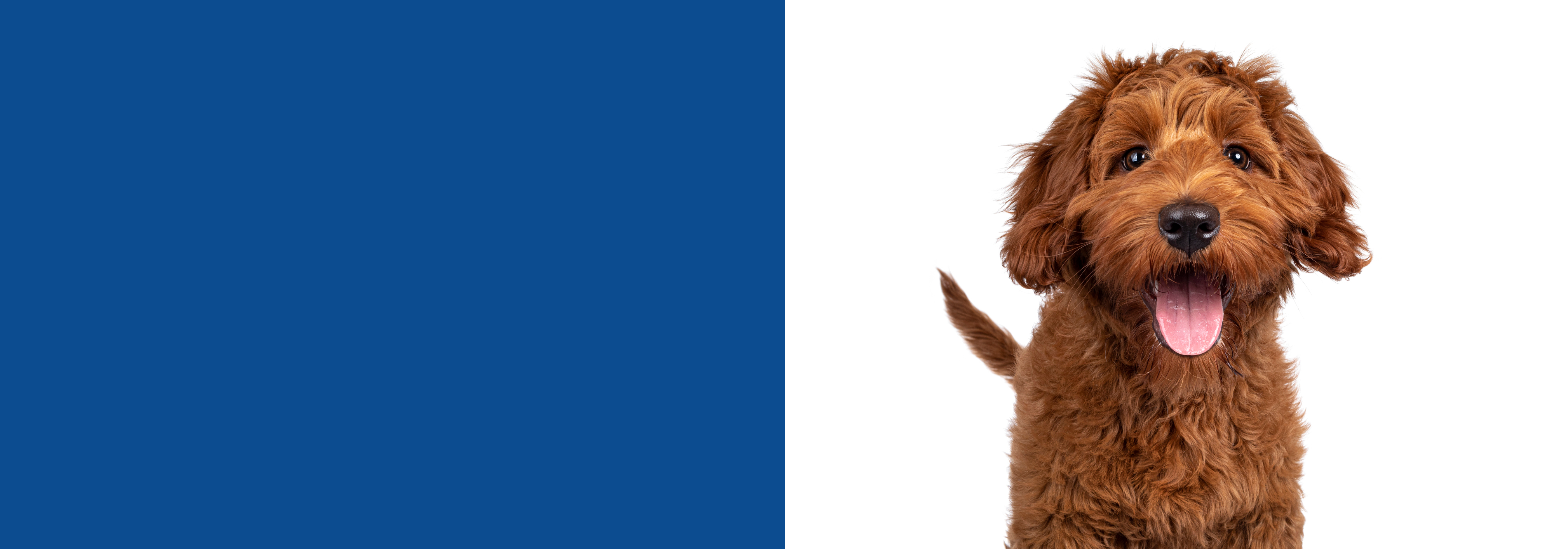
RapidRead Technology
- Artificial Intelligence (AI) is undeniably a part of our everyday lives. As veterinarians, we have a responsibility to educate ourselves about AI and the impact it will have on our practices and our patients’ care.
- This article explains machine learning in the context of veterinary imaging diagnostics and reviews how AIS is uniquely positioned to develop a strong and accurate AI product.
Article written by Diane U. Wilson, DVM, DACVR (August 2023, updated March 2024)
Artificial Intelligence at Antech Imaging Services
Machine Learning
In generative machine learning models (or algorithms), the machine can create solutions not previously programmed. One example is the chatbot, ChatGPT, which can learn, create and evolve conversations as it is being used. Whereas, in discriminative machine learning models, the machine resolves information only into classifications for which it is programmed. That is, it only identifies and categorizes whatever it was initially trained to identify and categorize. A discriminative learning model cannot evolve to something more. Currently, AIS uses only discriminative models for machine learning techniques. (Figure 1)
Regardless of whether you are optimistic or pessimistic, there is already significant use of AI in veterinary medicine. As in other areas of everyday living, the use of artificial intelligence can be transparent to the user. Whether the end user is a general practitioner, veterinary technician, or specialist, it may not be evident that artificial intelligence was employed, wholly or in part, to reach an objective. Sometimes, AI is used to assist another program in workflow or to assist a specialist in providing diagnostic information. Less commonly, AI performs an entire diagnostic test without human input.
Artificial Intelligence is Already Present in Veterinary Medicine
Machine Learning Must be Carried Out Responsibly
Massive amounts of data are needed to train a model on each finding. Without data, we cannot reliably assert claims of accuracy, sensitivity, and specificity. In people, we say one is an expert at a particular imaging finding when there is experience of at least 500 cases of that finding. Unfortunately, it is not so for a machine to learn.
At AIS, we have determined that we can be confident of the measured level of accuracy, sensitivity, and specificity of a model for a particular finding, when the model has encountered four to five thousand instances of that finding. For common findings, like a pulmonary bronchial pattern, the necessary number of cases to ensure accuracy can be easy to acquire. For less common findings such as diskospondylitis, it can take longer and require collaboration between multiple groups to gain the necessary number of cases to confidently train the model.
Domain experts (board certified specialists) are needed to train and measure the accuracy of each model. Radiologists (pathologists, cardiologists, dentists, etc.) spend hours labeling images and offering corrected data to be used to train, assess, and retrain the machine. Moreover, it is necessary to include a team of domain experts so that consensus can be reached, and the machine is not trained on the opinion of one individual. Third party investigations should be used to validate accuracy against known cases.
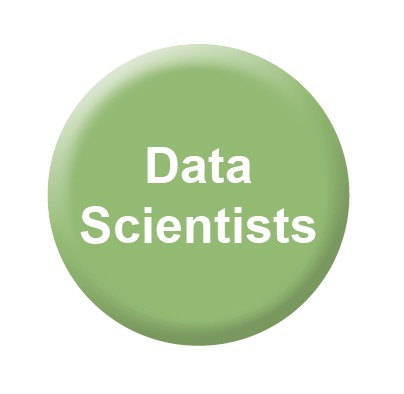
AI at AIS
Clinical application of a list of findings means several different findings can be present in any given report. Each individual finding may have an accuracy of at least 95%; however, consideration must be given to the report’s overall contribution of information to the case.
On the other hand, RapidRead is extensively trained in the findings listed in Figure 2. For example, if we are looking to learn if a patient has cardiac or lung changes that might contraindicate anesthesia or looking to determine if a patient with gastrointestinal signs is obstructed, then RapidRead is a very useful tool to speed expert level information to point of care in a matter of minutes (vs. hours to days for traditional radiologist reads).
Conclusion
Bibliography
“Colossus: The Forbin Project”. Universal Pictures. 1970. Retrieved from Prime Video.
Pu, Y., Apel, D.B. & Wei, C. Applying Machine Learning Approaches to Evaluating Rockburst Liability: A Comparation of Generative and
Discriminative Models. Pure Appl. Geophys. 176, 4503–4517 (2019). https://doi.org/10.1007/s00024-019-02197-1
Wilson, D.U., Bailey, M.Q., Craig, J. The Role of Artificial Intelligence in Clinical Imaging and Workflows. VRUS. 63:S1, 897-902 (2022)
https://doi.org/10.1111/vru.13157
Fitzke, M, et. al. OncoPetNet: A Deep Learning Based AI System for mitotic figure counting on H & E Stained whole slide digital images
in a large veterinary diagnostic lab setting. YouTube Video. https://www.youtube.com/watch?v=y_ZZUeW7Bvg&t=26s
Singh M, Nath G. Artificial intelligence and anesthesia: A narrative review. Saudi J Anaesth. 2022 Jan-Mar;16(1):86-93.
doi: 10.4103/sja.sja_669_21. Epub 2022 Jan 4. PMID: 35261595; PMCID: PMC8846233.
van Leeuwen, K.G., de Rooij, M., Schalekamp, S. et al. How does artificial intelligence in radiology improve efficiency and health outcomes?. Pediatr Radiol 52, 2087–2093 (2022). https://doi.org/10.1007/s00247-021-05114-8
Front. Hum. Neurosci., 25 June 2019 Sec. Sensory Neuroscience Volume 13 – 2019 | https://doi.org/10.3389/fnhum.2019.00213
Cohen, J, Fischetti, AJ, Daverio, H. Veterinary radiologic error rate as determined by necropsy. Vet Radiol Ultrasound. 2023; 1-12. DOI: 10.1111/vru.13259.